內容介紹 | |
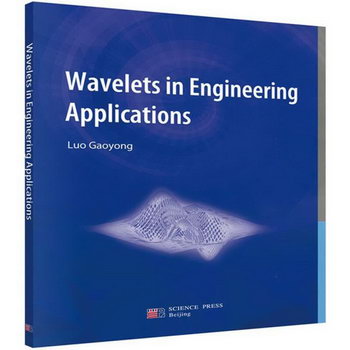
出版社:科學出版社 ISBN:9787030410092 商品編碼:68385558645 開本:16開 出版時間:2014-06-28 頁數:196 字數:400000 代碼:78
" 商品基本信息,請以下列介紹為準 | 商品名稱: | Wavelets in Engineering Applications | 作者: | Luo Gaoyong[編著] | 代碼: | 78.0 | 出版社: | 科學出版社 | 出版日期: | 2014-06-28 | ISBN: | 9787030410092 | 印次: | | 版次: | 5 | 裝幀: | 平裝 | 開本: | 16開 |
內容簡介 | 產品說明: 1. 本產品為按需印刷()圖書,實行先付款,後印刷的流程。您在頁面購買且完成支付後,訂單轉交出版社。出版社根據您的訂單采用數字印刷的方式,單獨為您印制該圖書,屬於定制產品。 2. 按需印刷的圖書裝幀均為平裝書(含原為精裝的圖書)。由於印刷工藝、彩墨的批次不同,顏色會與老版本略有差異,但通常會比老版本的顏色更準確。原書內容含彩圖的,統一變成黑白圖,原書含光盤的,統一無法提供光盤。 3. 按需印刷的圖書制作成本高於傳統的單本成本,因此售價高於原書定價。 4. 按需印刷的圖書,出版社生產周期一般為15個工作日(特殊情況除外)。請您耐心等待。 5. 按需印刷的圖書,屬於定制產品,不可取消訂單,無質量問題不支持退貨。
|
目錄 | PREFACE Chapter 1WAVELET TRANSFORMS IN SIGNAL PROCESSING. 1.1Introduction 1.2The continuous wavelet transform 1.3The discrete wavelet transform 1.4The heisenberg uncertainty principle and time-frequency decompositions 1.5Multi-resolution analysis 1.6Some important properties of wavelets 1.6.1Compact support 1.6.2Rational coefficients 1.6.3Symmetry 1.6.4Smoothness 1.6.5Number of vanishing moments 1.6.6Analytic expression 1.7Current fast WT algorithms 1.7.1Orthogonal wavelets 1.7.2Semiorthogonal (nonorthogonal) wavelets 1.7.3Biorthogonal wavelets 1.7.4Wavelet packets 1.7.5Harmonic wavelets 1.8Discussion REFERENCES Chapter 2SYSTEM MODELLING 2.1Introduction 2.2The underlying principle of Fourier harmonic analysis 2.3Autocorrelation wavelet algorithm 2.4Vibration model selection with FT and autocorrelation wavelet algorithm 2.5Coefficients estimation with least-squares algorithm 2.6Results and discussion 2.7Condition monitoring of bearing 2.8Concluding remarks REFERENCES Chapter 3CONDITION MONITORING 3.1Wavelet analysis 3.2Filter design and fast continuous wavelet algorithm 3.3Small defect detection of bearing 3.3.1Specific frequency ranges monitoring 3.3.2Significant and natural frequencies monitoring 3.4Concluding remarks REFERENCES Chapter 4PROCESS CONTROL 4.1Introduction 4.2Vibration and surface quality 4.2.1Theoretical calculation of surface quality 4.2.2Vibration during machining 4.3Adaptive spline wavelet algorithm 4.3.1Battle-Lemarid wavelet filter design 4.3.2Arbitrary fine time-scale representation 4.3.3Adaptive frequency resolution decomposition 4.4Methodology of experiment 4.5Results and discussions 4.5.1Experimental results 4.5.2Discussions 4.6Concluding remarks REFERENCES Chapter 5VIBRATION ANALYSIS 5.1Introduction 5.2Machining process vibration 5.3Wavelet algorithm with cross-correlation 5.4Experimental set-up 5.5Experimental results 5.6Discussion 5.7Concluding remarks REFERENCES Chapter 6AUDIO CODING 6.1Introduction 6.2DSP Implantation of lifting wavelet transform 6.3Embedded coding and error resilience 6.4Results of experiment and simulation 6.5Conclusions REFERENCES Chapter 7IMAGE QUALITY MEASUREMENT 7.1Introduction 7.2Wavelet analysis and the lifting scheme 7.3Image quality evaluation 7.3.1Image noise analysis 7.3.2Image sharpness analysis 7.3.3Image brightness analysis 7.3.4Image contrast analysis 7.3.5Image MTF anal |
摘要 | Chapter 1 WAVELET TRANSFORMS IN SIGNAL PROCESSING 1.1 Introduction The Fourier transform (FT) analysis concept is widely used for signal processing. The FT of a function x(t) is de.ned as +∞ X.(ω)=x(t)e.iωtdt (1.1) .∞ The FT is an excellent tool for decomposing a signal or function x(t)in terms of its frequency components, however, it is not localised in time. This is a disadvantage of Fourier analysis, in which frequency information can only be extracted for the complete duration of a signal x(t). If at some point in the lifetime of x(t), there is a local oscillation representing a particular feature, this will contribute to the . calculated Fourier transform X(ω), but its location on the time axis will be lost. . There is no way of knowing whether the value of X(ω) at a particular ω derives from frequencies present throughout the life of x(t) or during just one or a few selected periods. Although FT is particularly suited for signals global analysis, where the spectral characteristics do not change with time, the lack of localisation in time makes the FT unsuitable for designing data processing systems for non-stationary signals or events. Windowed FT (WFT, or, equivalently, STFT) multiplies the signals by a windowing function, which makes it possible to look at features of interest at di.erent times. Mathematically, the WFT can be expressed as a function of the frequency ω and the position b[1] 1 +∞ X(ω, b)= x(t)w(t . b)e.iωtdt (1.2) 2π.∞ This is the FT of function x(t) windowed by w(t) for all b. Hence one can obtain a time-frequency map of the entire signal. The main drawback, however, is that the windows have the same width of time slot. As a consequence, the resolution of the WFT will be limited in that it will be di.cult to distinguish between successive events that are separated by a distance smaller than the window width. It will also be di.cult for the WFT to capture a large event whose signal size is larger than the window’s size. Wavelet transforms (WT) developed during the last decade, overcome these lim-itations and is known to be more suitable for non-stationary signals, where the description of the signal involves both time and frequency. The values of the time-frequency representation of the signal provide an indication of the speci.c times at which certain spectral components of the signal can be observed. WT provides a mapping that has the ability to trade o. time resolution for frequency resolution and vice versa. It is e.ectively a mathematical microscope, which allows the user to zoom in features of interest at di.erent scales and locations. The WT is de.ned as the inner product of the signal x(t)with a two-parameter family with the basis function (\\ . 1 +∞ t . b 2 WT(b, a)= |a|x(t)Ψˉdt = x, Ψb,a (1.3) a .∞ (\\ t . b ˉ where Ψb,a = Ψ is an oscillatory |
" | | |